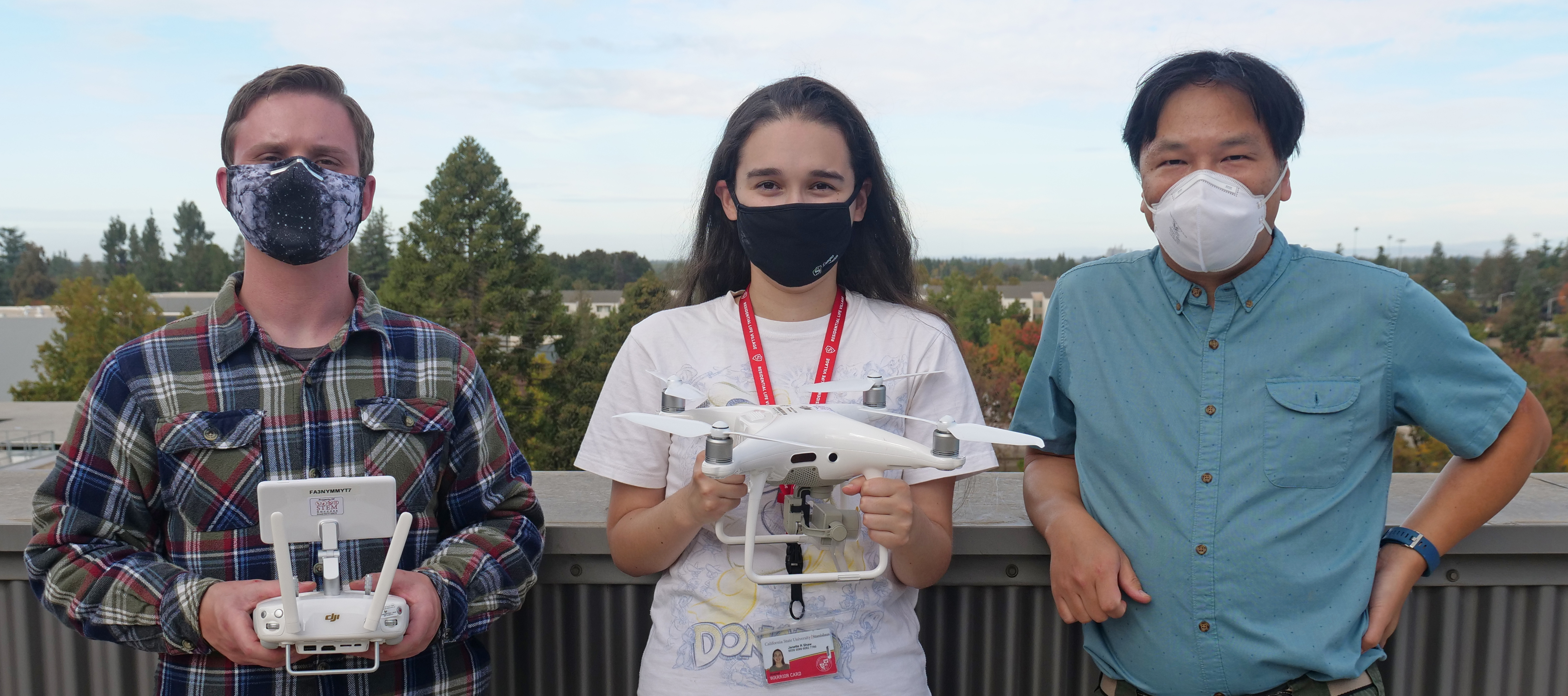
Research can have its roots anywhere, even on a friend’s front lawn. Just ask Wing To, an assistant professor of physics at Stanislaus State who went to an outdoor gathering last year and walked away with an idea for a research project that could speed up predictions of the atmospheric effects of California wildfires.
“It was at the start of the pandemic when people were hanging out on their lawns instead of indoors,” he said. “I was introduced to a couple of atmospheric scientists from the Lawrence Livermore National Lab. We were just having a beer on someone’s lawn and talking, and basically that is how the project started.”
The trio’s topic of discussion: how to change predictive modeling to produce quicker forecasts of the atmospheric effects of a wildfire before the pollution spreads over a wide area.
The traditional approach requires the calculation of complex mathematical equations, but atmospheric scientists are now exploring faster modeling methods using data science instead. With large forest fires happening more frequently, more historical data is available on how fires interact with the atmosphere. By using machine learning algorithms, also known as artificial intelligence (AI), scientists can use the data to model the effects of future fires.
By the time To left the get-together, he had an idea for a research project to address the issue and commitments from the two scientists to be the project’s expert advisers. He then secured two $5,000 Stan State grants — a Research, Scholarship and Creative Activity (RSCA) grant and an Innovate, Design, Excel and Asses for Success (IDEAS) grant—to fund the project.
Now underway, To’s project is collecting data from previous fire locations with the goal of using the data to upgrade simulation and modeling software that is already widely used by atmospheric scientists. If successful, the upgraded software will be able to produce accurate predictive models within days instead of the year or more that the process usually takes. This could lead to better public health protection because faster modeling could provide accurate, advance warnings of wildfire pollution as it heads toward communities.
To describes the project as “in its infancy.” His first step was to choose his starting point: the area of the Creek Fire that occurred in the Sierra National Forest in fall 2020. His next step was to start entering information about the existing plants and other material that could be consumed in a fire — creating a “fuel source map” — into a computer equipped with an AI program and coding acquired from Google. He made sure that his source of fuel information is a source that is updated frequently.
“The information about fuel that is often used is usually one or two years old. That is OK if you have a large wildfire in an area every 15 years, but nowadays because of climate change we are having wildfires in the same areas much more frequently,” he said. “We needed to find a way to update the fuel source map on a more regular basis.”
For a frequently updated source of information, To choose the European Space Agency’s Sentinel-2 satellite because it flies over the Creek Fire site every 10 days to take new images. Then he hired five Stan State students from the physics and computer science programs to scrutinize the satellite’s multi-spectral images and painstakingly tag each pixel to identify the type of fuel.
For details the students can’t get from the satellite images, To plans to take them and a drone to the site to collect more images. Ultimately, the students’ tags will train the artificial intelligence program to identify the fuel on its own, and the data will be updated every 10 days by frequent flyovers of the Sentinel-2 satellite.
One of those students is Taylor Whitney, a senior physics major and mathematics minor who is on track to graduate with a bachelor’s degree in spring 2022. He became involved with the project while taking an experimental physics class where he learned how to analyze data with Python software. After he was recruited to work on To’s research project, he attended a conference on wildfires and learned about the use of AI in wildfire research, which prompted him to teach himself machine learning with Python.
“Using AI to process and classify data is a powerful tool, but preparing data for the supervised machine learning algorithms is an extremely tedious and painstaking task,” Whitney said. “We have to look through the spectral data, create false color images and classify each pixel — or region — as a different kind of fuel source before we feed it into a computer program. The hope is that if we do this enough the computer will learn how to automate the process of classifying fuel sources more quickly and accurately than current methods.”
Also working on the project is Rommell Pennell, a senior physics major who expects to graduate in fall 2022 with a bachelor’s degree. He enjoys being involved with the project because it gives him an opportunity to work and experiment with a semiconductor device called a field programmable gate array (FPGA).
“I am interested in using an FPGA for research because I am able to build a simplified version of a processor such as those that may be in phones, cars and most electronics,” said Pennell, who likes to work with his hands to assemble circuits. “Through this project, I’m gaining in experimental research by assembling on a breadboard and programming the circuit using my computer.”
As work on the project proceeds, To hopes to hire a geography student and involve colleagues in the areas of geography and atmospheric, environmental, computer and fire sciences. He also hopes to obtain federal grants to fund the research that he believes will play an important role in helping Californians deal with the effects of future wildfires.
“When a fire happens, knowing where all the particulates are floating to, knowing where they will go and when they will get there within a day or two, that is pretty useful,” he said. “It is much more useful than knowing it a year later.”